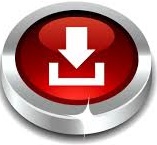
The leaf node’s output value is then used as a prediction for the input data. Making Predictions: Predictions are made by passing the input data down the tree, traversing the branches until it reaches a leaf node.Pruning involves removing branches of the tree that do not improve the predictive accuracy of the model. Pruning the Tree: Once the tree is fully grown, it is often pruned to reduce overfitting.Choosing the Best Split: At each stage of the tree-building process, the algorithm chooses the feature that best divides the data into the most homogeneous subsets based on a predefined criterion, such as mean squared error.
The goal is to create a tree with high predictive accuracy while remaining simple to understand.
Building Decision Tree: The decision tree is constructed by recursively splitting the training set into smaller subsets. The model is built using the training set, and its performance is evaluated using the testing set. Test Train Data Splitting: The dataset is then divided into two parts: a training set and a testing set. Data Collection: The first step in creating a decision tree regression model is to collect a dataset containing both input features (also known as predictors) and output values (also called target variable). Quick Overview of Decision Tree Regression ( Source) How Decision Tree Regression Works – Step By Step Finally, we will see an example of it using a small machine learning project that will also include DecisionTreeRegressor hyperparameter tuning. We will begin with a brief overview of Decision Tree Regression before going in-depth into Sklearn’s DecisionTreeRegressor module. In this article, we will look at a Decision Tree Regression tutorial using the Python Sklearn library. 5.6 Visualizing Regression Decision Tree with Graphviz Introduction. 5.1 GridSearchCV & Cross Validation in Decision Tree Regression. 5 Improving Results with K Cross Validation & Hyperparameter Tuning. 4.5 Splitting the Dataset into Train & Test Dataset. 4 Example of Decision Tree Regression in Sklearn. 3.1 Decision Tree Regressor Hyperparameters (Sklearn).
2.1 How Decision Tree Regression Works – Step By Step.2 Quick Overview of Decision Tree Regression.